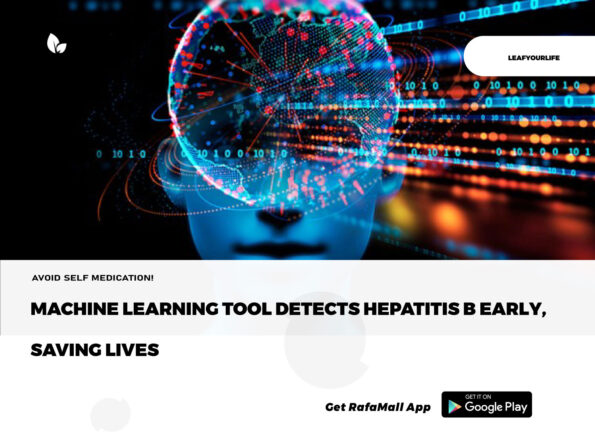
More than 296 million people worldwide live with hepatitis B, a potentially life-threatening liver infection caused by the hepatitis B virus (HBV). Most don’t know they are infected, so they don’t get medical care. Clinical care improves the patient’s outcome and can prevent them from infecting others.
Early detection of HBV-infected patients could therefore improve patient prognosis and stop transmission within populations.
The recommended test for HBV is an enzyme immunoassay. It detects the hepatitis B surface antigen – a substance that is a sign of the presence of the virus in the person’s body.
But these chemical tests are veryĀ expensiveĀ and need dedicated facilities. They are generally out of reach for people in low-resource settings, where laboratories are few and isolated. Clinicians in these settings work with limited resources againstĀ a silent killerĀ that may not show obvious symptoms for decades until the liver is severely damaged.
Part of the solution for public health challenges like this may lie in machine learning. This refers to the ability of computers to make sense of large amounts of information – and to build on their own “knowledge”.
We are among a group of researchers at the Australian National University who study machine learning and infectious disease. Our earlier research found that the prevalence of HBV in Nigeria was high (9.5%, where anything above 8% is considered high). And the levels of infection varied significantly across geopolitical zones.
Read more: Hepatitis B in Nigeria: fresh data to inform prevention and care
Access to affordable testing was a problem in the country. So we developed a tool to help clinicians detect hepatitis B infections earlier.
Using Nigerian patient data, we developed an algorithm that learns from the patient data, identifies patterns, and makes intelligent decisions to provide alerts and detection of a patient’s HBV infection status. The aim is to enhance clinical decision-making and improve patient outcomes. Enabling earlier care should give millions of people a better quality of life and help reduce HBV prevalence.
How did we do the work?
To build this tool, we worked closely with colleagues at the Nigerian Institute of Medical Research. They provided access to data from 916 anonymous patients, in an ethically approved manner. The institute is Nigeria’s foremost medical research institute and it hosts a dedicated hepatitis B clinic.
We used the results of normal blood tests that measure red and white blood cells, salts, enzymes and other blood chemicals, along with results of tests for hepatitis B. Routine blood tests can be very useful in facilitating early diagnosis if the subtle interactions between measurements can be spotted. Patterns of interactions may be a signal of disease. But it’s easy to miss them.
Using the data, we trained an algorithm to identify pathology markers that predict a patient’s HBV infection status. One reason machine learning is so powerful is that it does not require humans to tell the computer which features to identify. Our algorithm sifts through the data to find patterns that are common to patients with HBV infection and then match those patterns in people it has not seen before.
Once validated, the algorithm can be integrated into routine clinical workflow in a real-world clinical setting, as an intelligent decision support system. This will help detect HBV infections earlier, without resorting to expensive immunoassay.
What did we find?
For the 916 people in our study, our algorithm could reliably make an intelligent call to accurately predict those infected with HBV. Its discrimination threshold was 90% — indicating that the algorithm was highly accurate.
We then translated this into a user-friendly, web-accessible app to use in further studies. The decision support tool, Hep B LiveTest, was designed as a prototype.
The tool found that a combination of two enzymes, patient age and white blood cell count was the strongest predictor of HBV infection. The two enzymes are aspartate aminotransferase and alanine aminotransferase. When levels of these in the blood are high, it may indicate potential liver damage. Serum albumin, a liver function marker, was also identified as an important predictive marker of infection.
A study of Chinese patients showed trends similar to those suggested by our algorithm. Alanine aminotransferase and serum albumin were the most prominent predictors.
What’s next?
It is important to recognise the limitations of machine learning. Before a tool like this is put to work in routine clinical practice, it needs to be validated using diverse data.
Our machine learning tool was trained with data from Nigeria, so its performance may be limited to that setting. We are in the process of training our algorithm with more data from other sources and validating its robustness in other settings. This will inform how broadly applicable our algorithm is and how well it might work in other populations – particularly in settings with a low prevalence of hepatitis B infections.
Though our machine learning tool is only a first test, the results are highly encouraging. A person dies from viral hepatitis B every 30 seconds. We hope to put our system to work soon in the urgent fight against this vaccine-preventable disease.
We believe that machine learning has a role in enhancing the World Health Organization’s targets of eliminating viral hepatitis as a public health problem by 2030.
Busayo I. Ajuwon, Research Scientist, Australian National University
Brett A. Lidbury, Associate Professor
This article is republished fromĀ The Conversation AfricaĀ under a Creative Commons license. Read theĀ original article
Source: Allafrica
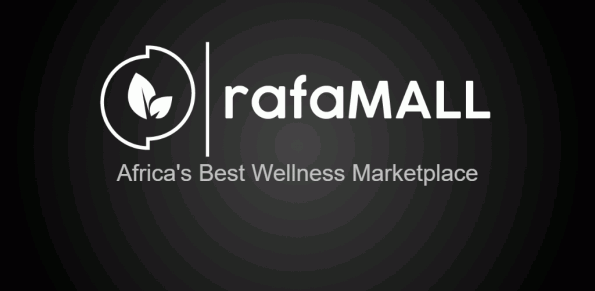